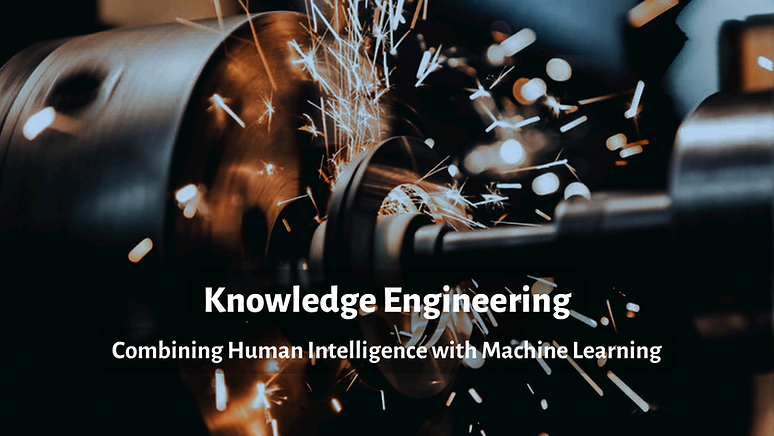
Knowledge Engineering Process
Asset Monitoring & Diagnostics (M&D) programs require a significant amount of experience to ensure detected anomalies are translated into actionable intelligence. A broad range of experience – from technical to non-technical skills – is accumulated over the course of a career. Industry is facing retirements at a sharply increasing pace and this is creating a challenge for M&D programs. For many organizations, the resources to hire and retain talent in preparation for this experience gap has not been prioritized. When experience is lost, the organization is faced with a gap that must be filled two ways:
-
Staff are expected to learn quickly
-
Technology is expected to fill some of the gap.
While human factors are key to addressing experience, there is an approach called Knowledge Engineering (KE) that can accelerate the capture of structured information in a format that can be digested by inexperienced staff. Recognized as part of the Fourth Industrial Revolution, the Knowledge Engineering process is an approach by which experience-based knowledge can be extracted, structured, and organized into a form that can be interpreted by a computer. It is important to note that KE-based solutions are not a replacement for experience, but rather a supplemental solution to assist with the experience gap. Additionally, KE-based solutions can supplement experience staff that are covering multiple roles in their organization.
Integral Analytics leverages engineering know-how, asset understanding, and collaboration between knowledge engineers and subject matter experts to build asset analytics capabilities. Our approach to a typical project involves the following steps:
Document asset operating principles
Asset operating principles are documented to formalize how your asset operates on macro and micro levels. For example, a Main Feedwater Pump is evaluated as a whole (macro), as its individual systems (lubricating oil, seals, bearing wear, etc.), and the feed water and support systems act upon the main feed water pump (lubricating oil system cooling water, motor/turbine parameters, etc.).
Perform a Failure and Modes Effects Analysis (FMEA)
The FMEA includes evaluating each failure mode for its individual degradation mechanism and influence. Then, degradation mechanisms and influences are evaluated for a sensor or group of sensors that may detect each mechanism or influence based on individual detectability. Finally, an analysis method is identified for each sensor – degradation mechanism pair.
Assess instrumentation and data availability
Once the ideal sensor and analysis set is identified, a gap analysis is performed. This gap analysis determines what can be implemented in the near-term and what needs to be implemented in the future. The gap analysis identifies not just sensors needed, but also data requirements.
Translate FMEA into a real-time diagnostic model
This is where all of the above is put together into a detailed, staged plan, building the complete model for your asset.
Evaluate historical data for analytic model design
Historical data is evaluated for evidence of steady state, transient, and abnormal conditions. This is used to create a repeatable model were, when recognized by the system, warnings with specific messaging can be developed, capturing knowledge from subject matter experts (SMEs).
Assess data analytics and diagnostic models with SMEs
In the final stage of this approach, SMEs evaluate each data set – condition and provide their input for the final assessment. The knowledge captured here will advise less- and inexperienced analysts and operators during future analysis, providing more than that “gut feeling” approach to machine diagnostics.
The above project steps can typically be accomplished in 6-8 weeks per asset (e.g., system/component) and yield results that can be reused for similar assets.
The value in a Knowledge Engineering approach can be determined through opportunity cost and direct savings. Direct savings are two-fold. First, the time between attention and decision is immediately reduced, allowing for faster time-to-repair, lessening the threat of catastrophic failure. Second, the chance of making an incorrect decision is lessened based on captured and documented direct experience, rather than guessing at a diagnosis. The opportunity cost is recovered by allowing the operator or analyst to focus on a greater number of alerts, rather than attempting to evaluate a situation that one may not be knowledgeable.
Knowledge Engineering, enabling artificial intelligence to imitate how a person may react in different situations, can lead to direct and indirect savings by capturing first-hand knowledge from experienced personnel and codifying that experience into your diagnostic systems. With the Integral Analytics approach, through a repeatable process, you can increase efficiency and reduce risk, putting your M&D program leaps and bounds above your competition.
Download the Knowledge Engineering guide or contact us today for more information.
